Research Proposal: Optimizing User Experience in the Era of Streaming Overload—Exploring Interface Design, AI Integration, and Cross-Platform Solutions for Content Discoverability
I. Introduction: Navigating the Digital Flood—Why UX Matters More Than Ever in Streaming Services
In recent years, the exponential growth of digital streaming services has profoundly reshaped the way audiences consume media. With platforms such as Netflix, Hulu, Disney+, Amazon Prime Video, and many others competing for viewer attention, the modern media landscape is saturated with an overwhelming amount of content. While this abundance has increased viewing options, it has also led to the unintended consequence of cognitive overload, complicating the user journey from intent to engagement. This paradox—more content, yet more friction in accessing it—has given rise to what scholars and industry leaders now term the “streaming search crisis” (Shapiro & Ranchet, 2025).
An expanding body of academic and industry research underscores the growing importance of user experience (UX) as a central driver of user engagement, satisfaction, and retention in digital environments. As Hassenzahl (2010) argues, UX is not merely about technical functionality or interface design but involves holistic experiences, including emotional resonance, perceived control, and the alignment of interface design with personal goals and preferences. Poor UX design in the streaming world manifests as information overload, frustrating navigation, ineffective recommendation algorithms, and interface inconsistency—often leading to what is known as “decision fatigue” (Lim et al., 2022).
Shapiro and Ranchet (2025), in The Media Odyssey podcast, delve into these concerns and offer a critique of the current state of streaming user interfaces. They highlight how fragmented app ecosystems, unintuitive navigation schemes, and poor algorithmic transparency are actively degrading the user experience. Their insights align with the findings of Lee et al. (2021), who demonstrated that viewers frequently abandon platforms not due to content scarcity but because of challenges in discovering and accessing that content. These insights emphasize the importance of understanding both the psychological and technological dimensions of UX.
While personalized recommendation systems powered by artificial intelligence (AI) offer promising avenues for improved discoverability, these systems are only as effective as the interfaces through which users interact with them (Zhao et al., 2020). The interface must bridge the gap between algorithmic output and user intuition. An innovative response to these challenges is the development of Swipe Finder, a swipe-based, cross-platform content discovery tool introduced in the podcast. Designed to mimic familiar, intuitive interaction models from dating apps, Swipe Finder centralizes user experience and presents a unified discovery platform across multiple services. Its white-label potential and business model open avenues for monetization while centering on a seamless user journey.
This research seeks to explore the critical shortcomings in current streaming service interfaces and to evaluate emergent models like Swipe Finder that aim to resolve these user-centered challenges. By drawing upon interdisciplinary fields such as human-computer interaction (HCI), media psychology, and digital interface design, this study aims to generate actionable insights into how interface design and AI-driven personalization can be synergized to deliver meaningful, engaging, and frictionless user experiences in a competitive and content-rich digital ecosystem.
II. Central Research Question
How can user experience (UX) design in streaming platforms be optimized to improve content discoverability, reduce user frustration, and enhance viewer engagement in the context of content overload?
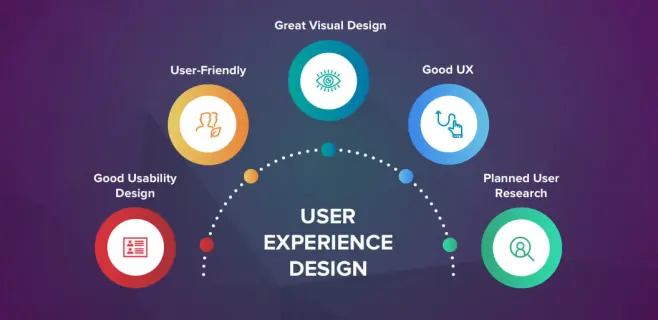
III. Research Objectives
- To analyze the key UX pain points experienced by users across major streaming platforms (e.g., Netflix, Hulu, Disney+, Amazon Prime Video).
- To evaluate the effectiveness of existing content discovery mechanisms, including search engines, content carousels, and AI-based recommendation systems.
- To assess user perceptions and behaviors associated with choice overload, interface inefficiencies, and disengagement.
- To examine innovative, third-party solutions such as Swipe Finder as a case study for improving cross-platform discoverability and user satisfaction.
- To develop a set of evidence-based design principles and guidelines for streaming service UX improvements that align business and user goals.
IV. Methodology: A Mixed-Methods Approach to Streaming UX Evaluation
This research will adopt a comprehensive mixed-methods approach that integrates both qualitative and quantitative methods to explore UX issues from multiple perspectives and generate triangulated findings.
- Literature Review
A systematic literature review will be conducted to understand the theoretical foundations and recent developments in UX, HCI, digital media behavior, and AI-powered personalization systems. Scholarly sources will be drawn from databases including ACM Digital Library, Scopus, IEEE Xplore, and ScienceDirect. The literature review will serve to establish the conceptual framework of the study and identify key gaps in current research. - User Experience Audit
An evaluative UX audit of five leading streaming platforms will be conducted using heuristic evaluation techniques. Metrics will include Nielsen’s 10 usability heuristics, visual hierarchy analysis, navigation flow diagrams, and the System Usability Scale (SUS). Comparative scores will be generated to highlight platform strengths and weaknesses in UX design. - User Surveys
A structured digital questionnaire will be administered to a sample of at least 100 streaming users. The survey will collect quantitative data on:- Perceived ease of navigation and content discovery
- Satisfaction with personalization features and algorithmic suggestions
- Frequency of content abandonment due to interface complexity
- Preferred discovery tools (search, curated lists, algorithmic suggestions)
- User Interviews
In-depth, semi-structured interviews will be conducted with 10–15 participants to collect qualitative data on their lived experiences with streaming interfaces. Topics will include emotional responses, trust in recommendations, discovery fatigue, and interaction flow preferences. Interviews will be transcribed and analyzed using thematic coding. - Case Study Analysis: Swipe Finder
Swipe Finder will be examined as a prototype or deployed product (depending on availability) through a case study methodology. This will include interface walkthroughs, user testimonials (if accessible), and interviews with developers or product managers. The analysis will evaluate Swipe Finder’s value proposition, interface design logic, integration potential, and monetization model. - Data Analysis
Quantitative data will be analyzed using SPSS or R Studio. Descriptive statistics will identify trends, while inferential tests (e.g., t-tests, ANOVA) will compare UX ratings across platforms. Qualitative data from interviews will be coded thematically using software such as NVivo or MAXQDA to extract user sentiment, behavioral patterns, and design implications.
V. Key References
- Hassenzahl, M. (2010). Experience Design: Technology for All the Right Reasons. Morgan & Claypool Publishers.
- Lee, M., Choi, H., & Kim, J. (2021). “Understanding the Paradox of Choice in Video Streaming Services.” International Journal of Human–Computer Interaction, 37(8), 771–784.
- Lim, S., Yoo, S., & Park, J. (2022). “User Experience in Digital Media: The Role of Navigation and Personalization in Streaming Platforms.” Computers in Human Behavior Reports, 5, 100170.
- Zhao, X., Zhang, Y., & Wang, C. (2020). “Interface Design and AI-Powered Recommendation in Streaming Services: A UX Perspective.” Journal of Media Technology and Innovation, 7(3), 145–160.
- Shapiro, E., & Ranchet, M. (2025). Fixing TV’s UX: Solving the Streaming Search Crisis. The Media Odyssey Podcast, Episode 13.
Note: Additional sources and updated data sets will be incorporated as the project progresses into the data collection and analysis phase.
Leave a Reply